CompInterp
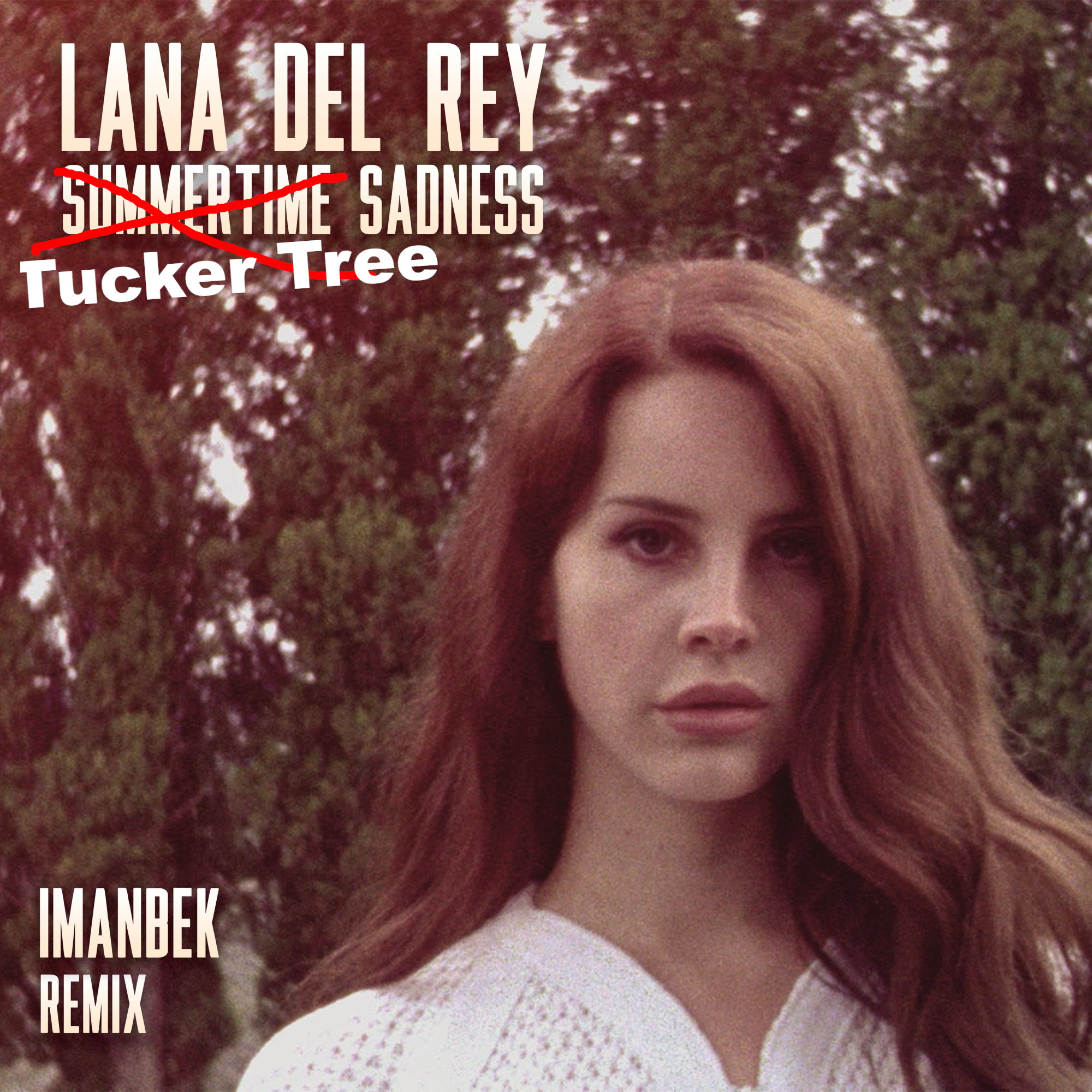
The CompInterp approach to interpretability treats weights and data as a unified modality to provide a compositional perspective on model design, analysis, and manipulation. By combining tensor and neural network paradigms, our $\chi$-nets pave the way for inherently interpretable AI without sacrificing performance.
$\chi$-nets are compositional by design, both in how they are built and in the representations they learn. Their architecture enables mathematical guarantees and weight-based subcircuit analysis, grounding interpretability in formal (de)compositions rather than post-hoc activation-based approximations.
We’re currently scaling CompInterp methods to CNNs and transformers by leveraging their specialised low-rank structure. Learn more about it in our latest talk!